How Accurate is Unacast Data?
By the Unacast Data Ombudsmen Team
To deliver the highest-quality offerings possible, the core of Unacast’s philosophy about location data and human mobility insights is accuracy.
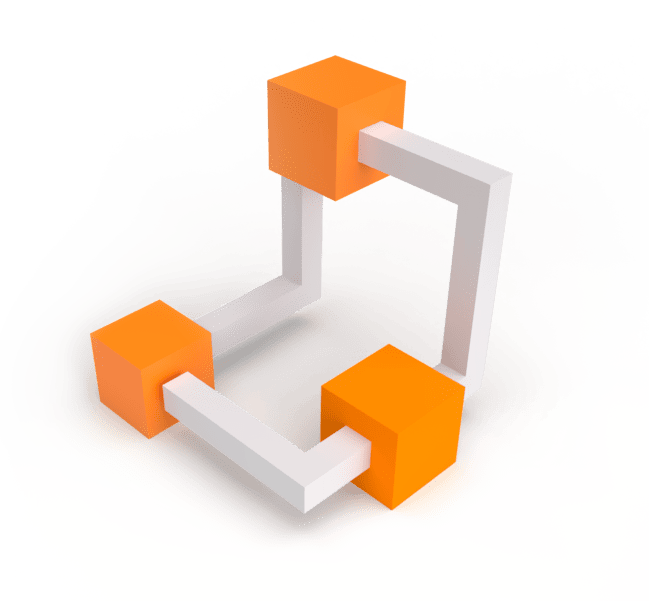
By the Unacast Data Ombudsmen Team
To deliver the highest-quality offerings possible, the core of Unacast’s philosophy about location data and human mobility insights is accuracy.
Therefore, the purpose of this work is to determine and demonstrate the accuracy of our data. The sample here is based on home areas defined in our "Home & Work" data package and is not restricted in time. Ergo, the data represents all home areas in Unacast data history. It is also important to note that the analyses here are NOT based on device-level demography data, and are solely based on applying census demographics from the census block group level on Unacast data. Analyses of Unacast data are performed regarding four different research questions:
To investigate geographical bias in Unacast data, we calculated the observed proportion of Unacast data within each geographical area. In addition, we do the same for census data, which gives us the expected proportion. A bias in geographical sampling would then be apparent in the difference between the observed and expected proportions. In more detail, if this difference (namely sampling bias) is >0, we oversample from a specific area. If sampling bias however is <0, we undersample from an area, respectively.
The results show that Unacast data is very well representing the census proportions. As can be seen in Figure A, the correlation coefficient between the number of residents per state in the Unacast sample and number of people living in this state is almost perfect (r = .99).
Additionally, Figure B shows that sampling bias varies only from -1.89 to 1.84 percentage points, with the average being 0.02. This indicates that Unacast data is very close to the proportion of the census population.
Plotting those sampling biases on a map (Figure C) shows that Unacast data is slightly under-sampled for California and slightly oversampled for Texas and Florida.
For this analysis, we are not using device-level demographics but instead inferring from Census. Because our supply might contain more data from higher-income or lower-income neighborhoods, the goal here is to investigate potential bias towards areas with certain income groups in Unacast’s sourced location data. To do so, we used census income data for each block group in the US. Unacast data was split within each block group into the income ranges and proportions determined by the census data. Aggregating those fractions for the whole US or on the state level indicates whether or not there is an income bias in the Unacast sample.
The result shows that Unacast data proportionally represents higher-income neighborhoods. This can be seen in Figure 4, where the value of each bar represents the proportion in Unacast data and the red dot the proportion in Census data. The graph illustrates that Unacast data is mostly coming from medium-high income ranges ($60k-150k), and that extremely high income ranges (>$150k) are proportionally less represented — nonetheless we are under-representing those incomes compared to the Census data.
Investigating Unacast data on the state level (Figure 5) shows that in certain states (e.g., Maryland or New Jersey) people of higher incomes are over-represented. However, in other states, Unacast is over-representing people of lower income (e.g., Mississippi or Arkansas). Nevertheless, even those “over-” / “under-”representations are quite close to the expected representations by census data.
Similar to the investigation of income, we used census block group data to calculate whether Unacast data is over- or under-representing certain age groups.
The results show that Unacast data evenly represents people of different ages. Unacast data is evenly distributed across age groups spanning from 20 to 65 years (Figure 6). Younger groups (< 20 years) and older groups (> 65 years) are, however, less represented in Unacast data.
Overall, the data is very close to the census data (red dots). Only for the very young (< 20 years) and the very old (> 80 years) age groups, Unacast data is meaningfully diverging from the census.
On the state level, Unacast data is more representative of younger people than older people. Unacast data is, in certain states (e.g., Pennsylvania or Maine), evenly distributing age; whereas in other states Unacast is more over-representing younger people (e.g., California or Utah).
Similar to the investigation of income, we used census block group data to calculate whether Unacast data is over- or under-representing the genders.
The results show that Unacast data evenly represents different genders. On the state level (Figure 8) it appears that in most states Unacast data is slightly more representing men than women but this gender bias reaches a maximum of only 0.09%. Intriguingly, North Dakota shows the opposite effect, in which Unacast data represents more women than men (by up to 0.2%).
This study helped us analyze and monitor our data, which is the foundation for all of our aggregated data products and Human Mobility Insights. When compared to census data, Unacast data is overwhelmingly accurate in representing geography, income, age, and gender.
Going forward, we will repeat these analyses at regular intervals, using the results to diagnose and correct for the cause of geographic bias and data inaccuracies — ensuring that the products that reach our clients are of the highest possible quality.
Didn't find the info you were looking for? Don't worry, you can search the entire site to easily find what you want.